Geochemical
data: heavy metals in till.
The dataset
includes 1407 samples from till (moraine) over the area of 100
x 100 kilometers. Variables are: X, Y (sample coordinates, m),
Cu (copper), Co (cobalt), Ni (nickel), Pb (lead), V (vanadium),
Zn (zinc) (contents, ppm=parts per million). Glacial till is a
mixture of rock material, and the levels of metals in till represent
the natural geochemical background. There are three main rock
types present within the area: granites (70% of the area), acid
volcanic rocks (20%), and basic rocks (10%).The concentrations
of heavy metals vary from 0 to 50-200 ppm (Figure 1). The freqency
distributions of values are positively skewed, with varying number
of outliers for different metals.
The first
step is to identify and deal with outliers. After that, we are
interested in identifying and quantifying some "multivariate
fingerprints" (associations of metals) which are related
to known bedrock types. Normally the geochemical data would be
transformed (or outliers removed) in order to proceed with uni-
and multivariate statistical analysis. The outliers may, however,
contain a lot of valuable information. Analysis with GIS techniques
assume interpolation of the point data, and discovering the relations
between elements would mostly be based on visual displays.
At first
look, the outliers can be easily identified, and there are no
visible clusters in the data (Figure 1). From the scatterplot
display one can see that most elements are more or less positively
correlated with each other, except for Pb (Figure 2).
Figure
1.
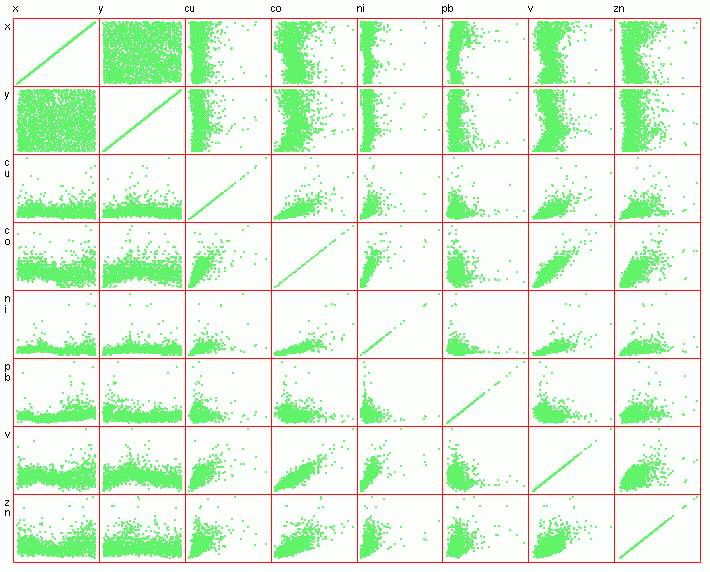
Figure
2.
The outliers
can be brushed to see if there are any atypical ones. For example,
looking at the highest values for Ni, the brushed samples (Figure
3) are located mostly in the eastern part of the area (Figure
4) and certainly belong to the same type of rock, which is enriched
with Ni, Co, Cu, V and Zn (basic rocks, confirmed by a bedrock
map).
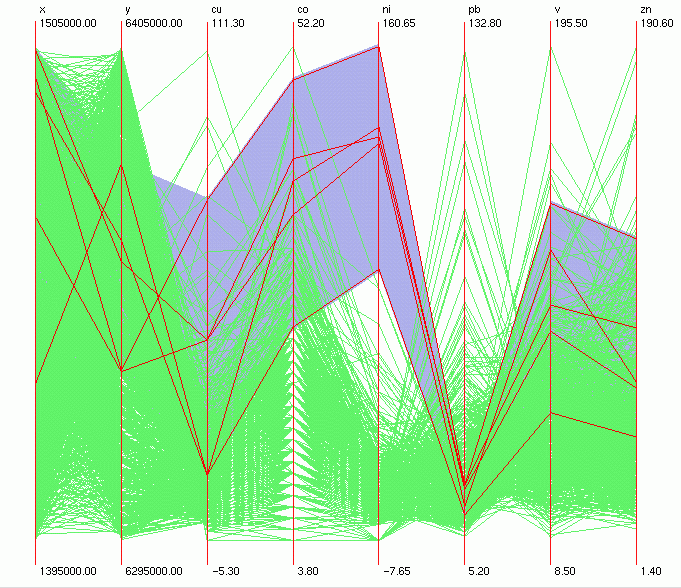
Figure
3.
Figure
4.
In order
to study the natural geochemical anomalies within the area, we
can limit the ranges of concentrations of interest to the last
10% of the samples in the high range. For example, we are interested
in metal Pb and its relations with other metals (the scatterplots
showed no or weak correlations). The 90th percentile value for
Pb is about 35 ppm. Brushing the samples with Pb levels equal
or higher than 35 ppm (Figure 5) shows that they are concentrated
in the S-SE and NE of the area (Figure 6), and their "multivariate
fingerprint" is a bit unclear (Figure 5). Pb and Zn seem
to be correlated (that indicates some common source), but the
relations with other elements are not as easy to interpret. For
example, we can see that Pb and V have several kinds of relations
(both positivly and negatively correlated samples), and we can
try to separate those.
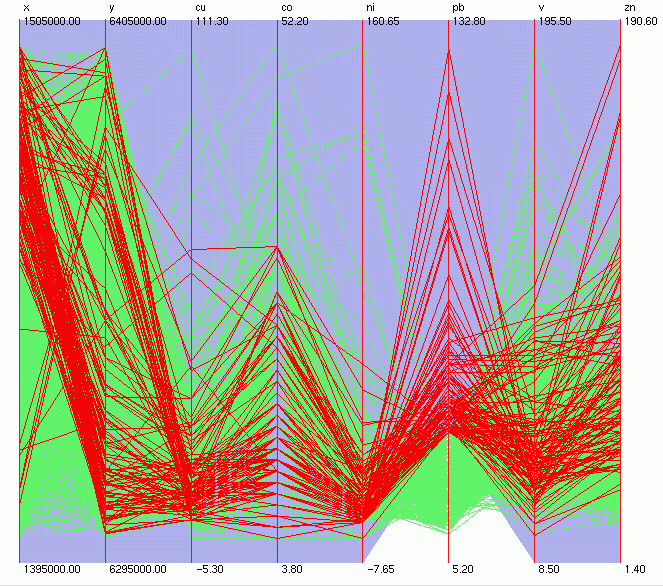
Figure
5.
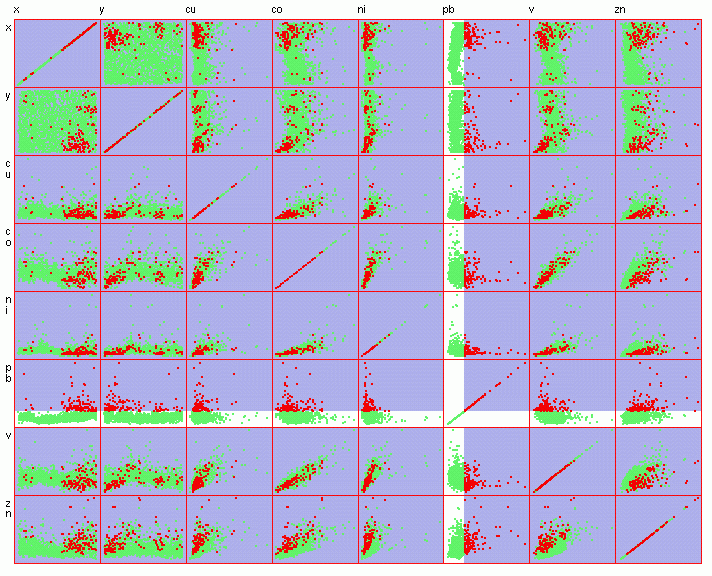
Figure
6.
We first
brush the samples that have the highest concentrations of Pb,
together with low V levels (Figure 7). The scatterplot display
indicates that most of those samples (Figure 8) belong to the
above-mentioned S-SE cluster ( Figure 6), and contain relatively
low levels of Co (less than 20 ppm).
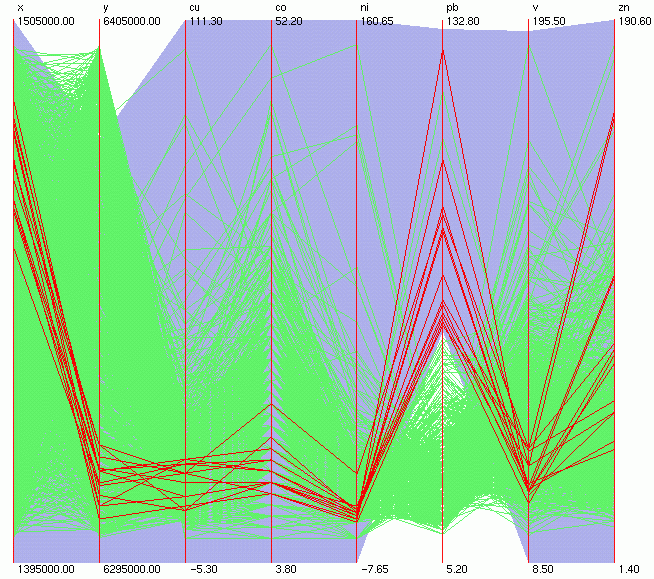
Figure
7.
Figure
8.
Now, let's
select all the samples with V equal to or higher than 75 ppm (the
90th percentile). The scatterplot shows a main cluster of points
in the E side of the area (Figure 10). We can see that there is
strong positive correlation between V and Co (Figure 9). For simplicity,
we do not consider the elements Cu, Ni and Zn.
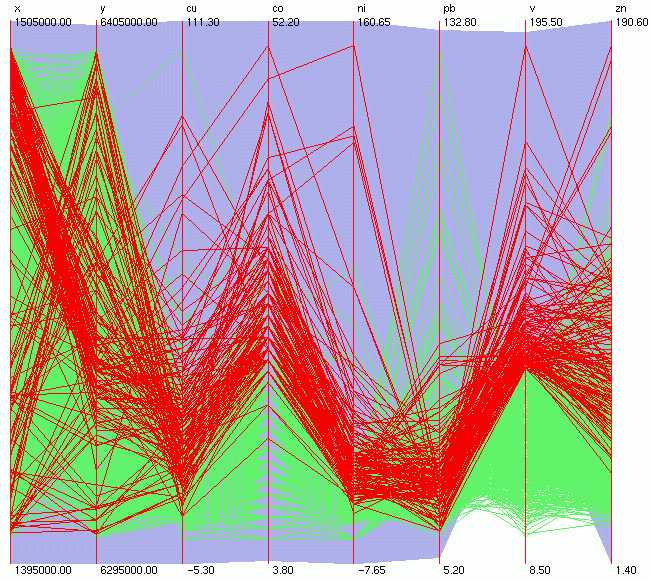
Figure
9.
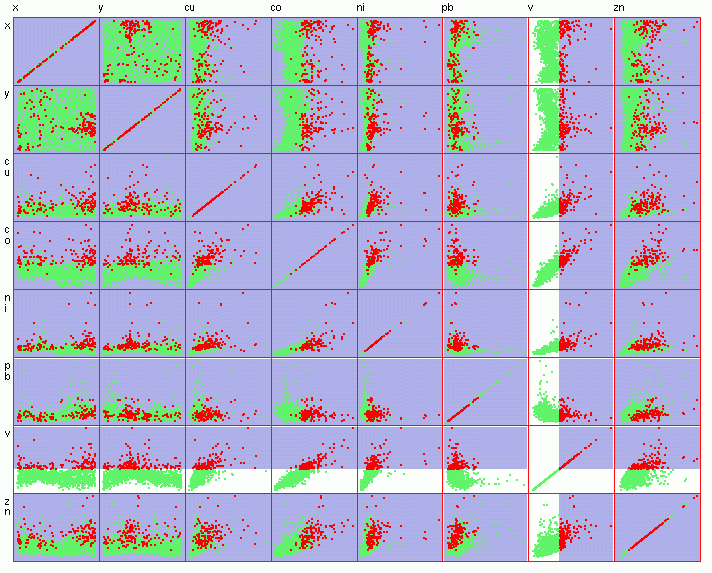
Figure
10.
From the
parallel coordinates display above (Figure 9) we can notice a
small cluster of samples with nearly parallel lines between the
Pb and V axes. Those points have both Pb and V over their respective
90th percentile values (Figure 11, Figure 12) and belong to both
the S-SE (Figure 6) and E (Figure 10) clusters. Looking closer
at the first row (or first column) in the scatterplot matrix (Figure
12) we can tell that this is rather an overlap of the two anomalies
than a new multivariate fingerprint, because the values of V and
Co differ slightly in the two clusters.
Figure 11.
Figure 12.
Data courtesy
of SGU (the Geological Survey of Sweden).
Katrin Grunfeld
katring@geomatics.kth.se
|